Cutting-Edge Research Roundup
Perusing the academic repository Arxiv.org to look for the future of AI.
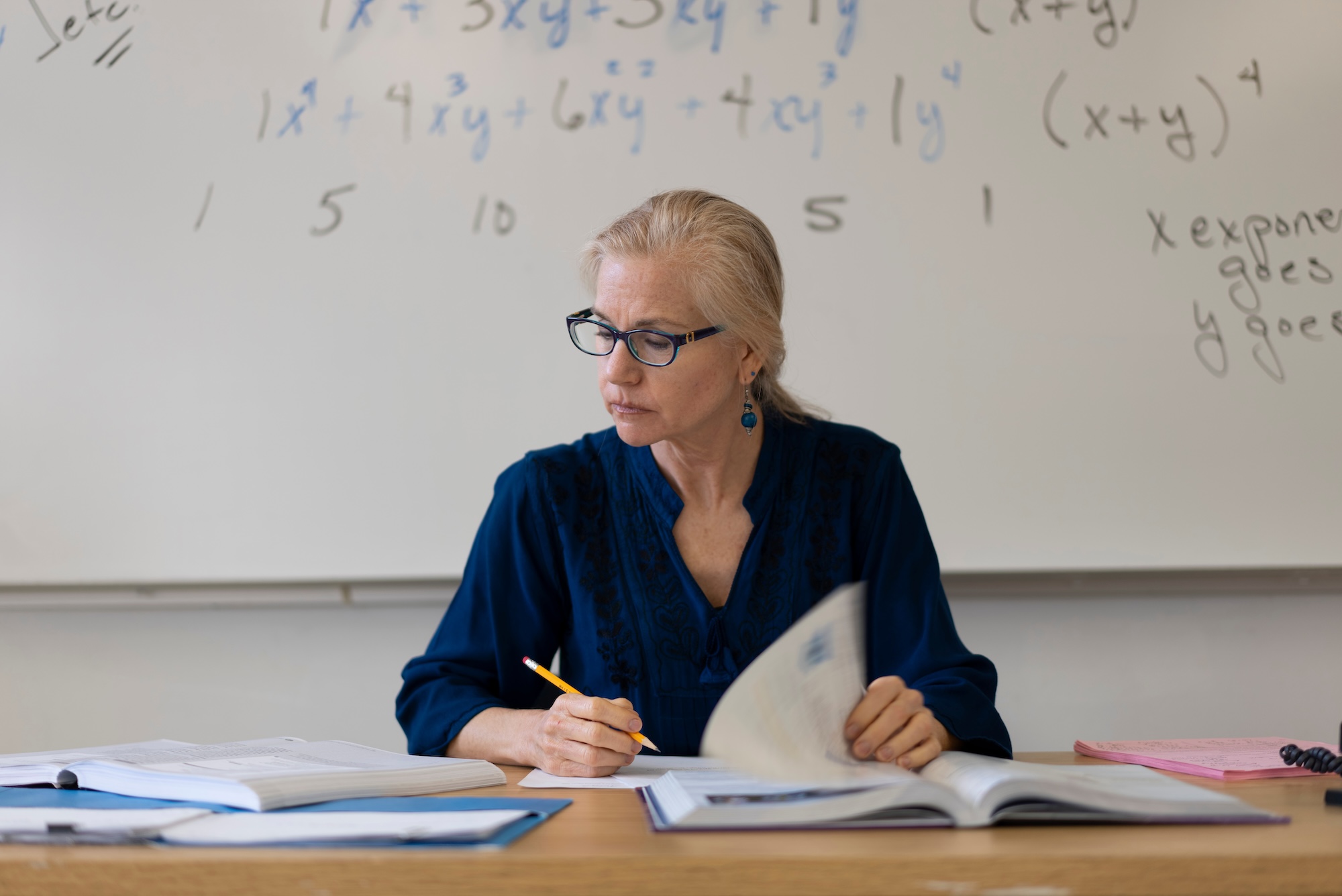
She’s grading AI papers and very few get good grades.
If you want to know the true bleeding edge of technology, a good place to look is always Arxiv.org, from Cornell University. It’s a huge repository of academic research in the sciences, often published before any official peer-review process. As you might expect, the Computer Science/Artificial Intelligence category is absolutely on fire right now—sometimes hundreds of papers are published per day. I’ve started skimming them, just to read the room, picking up on titles, topics, and abstracts.
Looking through the last week or so—about a thousand papers—you see that AI is being used in myriad ways, such as:
- combatting illicit firearms trafficking
- Alzheimer’s disease prediction
- architectural space layout planning
- exploration of gene expression data
- Arabic handwriting recognition
- diagnosis of acute aortic syndrome
- automatic music transcription
- Yemeni currency detection
- breast cancer diagnosis
And a few patterns jump out:
- There’s a lot of basic research and alignment with pre-existing work—stuff like “multi-agent generation via evolutionary algorithms,” bringing the gee-whiz of a decades ago into the gee-whiz of today. Topics like handwriting recognition or music transcription fit squarely into the last seven decades of AI research. This is academic bread-and-butter stuff: “Using new technique B, we were able to achieve different results than using old technique A.”
- There’s a lot of foundational organizing work going on—rankings of different language models, descriptions of fundamental methods, pointers to useful math, and so forth. The new, LLM-based twist on AI is becoming its own discipline, so it needs a taxonomy and a way of structuring itself. Decades of standards-body meetings await! This is the sign of an industry finding itself and building up the infrastructure it needs.
- There is some investigation into the bias and social problems of AI (a good example: “QueerBench: Quantifying Discrimination in Language Models Toward Queer Identities”). Not a ton of papers, though, given how prevalent this aspect of AI seems to be in the news. This also makes sense, because this kind of work gets less funding, from fewer sources. (It’s also vaguely possible that such papers are not being submitted to Arxiv because they’re aimed at a social sciences community, rather than a hard sciences one.)
The big thing I learned is that what pops up, more than anything, is medicine. It’s obvious that there’s just a ton of money going into medical AI research (and indeed there was a big article about this in Nature in late 2023). This gets less coverage than other AI stories, but it makes sense! Medical research is well-funded, and medicine has vast data and analysis challenges. If you work in technology long enough you’ll meet tons of people who work inside of hospital systems—there’s a lot of product work, experimentation, and huge data challenges in health science.
It also points to an interesting future, one where AI is less about what new tricks the bots have learned and more about ensuring the accuracy of breast cancer diagnoses. The accuracy issues that Google or OpenAI keep shrugging off won’t fly with medical applications—you’d be looking at the biggest lawsuits ever if you didn’t rein it in. You have to wonder if true AI safety will come out of Silicon Valley, or out of some giant hospital group. If you want to work in AI ethics and safety it might be more worthwhile to apply to work for a doctor in their lab than for Google.
Doing little mini-surveys of the literature like this rarely produces a ton of surprises, but it’s good context, and it’s wild to see just how much is happening, across so many different fronts. By the time this is published at least a hundred new papers will be published on AI alone—check them out if you’re curious to see a whole discipline operating across the globe.